Description
Currently, facial and body movements are better controlled and categorized by neural systems than any existing technical framework.
Our lab studies the computational principles underlying the control and categorization of body movements in biological systems and transfers them to technical applications, e.g., in computer graphics, humanoid robotics, or biomedical engineering and rehabilitation, where modeling human movements is becoming increasingly important for technical success.
Researchers
Current Projects
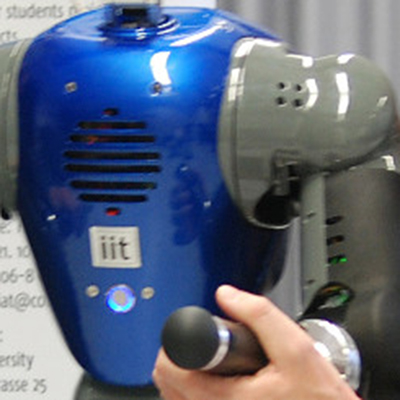
Modeling of human robot interaction and use of humanoid robots for rehabilitation training
The control compliant robots in interactive tasks, or even in joint tasks with multiple interacting humans and robots is a challenging problem. It is adressed in the EC H2020 project COGIMON by a highly interdisciplinary approach, combining the expertise form groups in neuroscience and robotics engineering. One interaction scenario of this type is also the training of patients by playing an interactive ball game with a humanoid robot. We used biologically inspired algorithms for movement syntheses to control humanoid robots and for training of coordination skills in ataxia.
Read more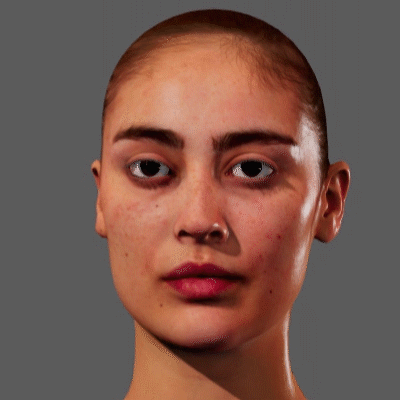
Deep Gaussian Process models for Real-Time Blendshape Prediction on GPU
Modeling virtual character blendshapes via approximate inference in real-time on GPU. This implementation is realized as plugin for Autodesk Maya and Unreal Engine 5.
Read more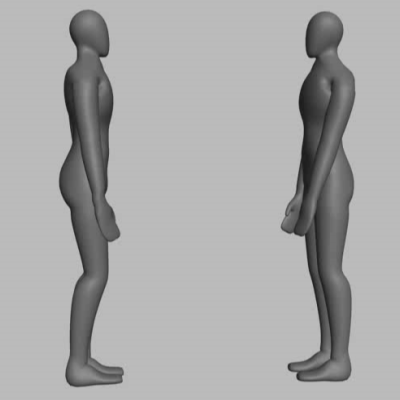
Probabilistic models for the online-synthesis of emotional and interactive full-body motion
Generative probabilistic models of interactive and stylized human motion are applicable in a variety of fields. On the technical side, such models are useful in computer animation, or motion recognition and emotional feature analysis. This work was done partly as parts of the EC FP7 projects TANGO.
Read more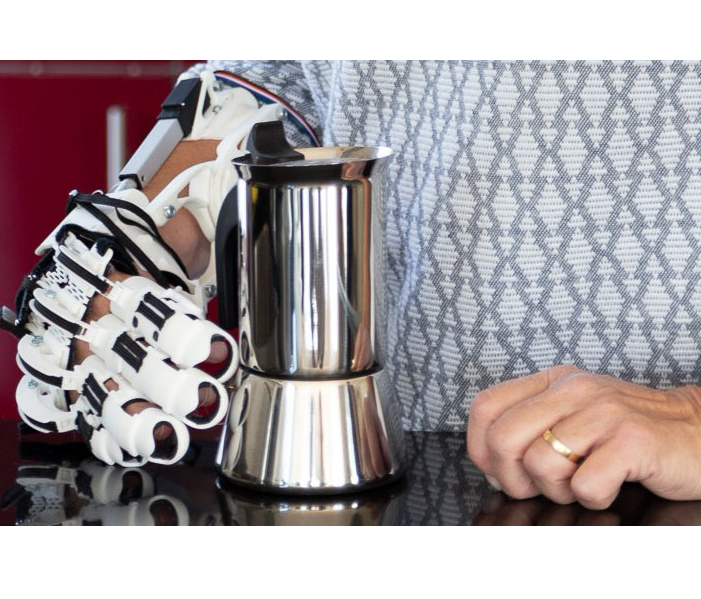
Online Controllable Models of Complex Body Movements for Biomedical Applications
Robots driven by signals from the nervous system represent a promising approach to improving the autonomy and the quality of life of people with disabilities, following, for instance, stroke or spinal cord injury. The aim of the KONSENS-NHE project is the development of a non-invasive, neurally-controlled exoskeleton that may be used in everyday life to compensate for the loss of hand function in people with disabilities.
Read moreFinished Projects
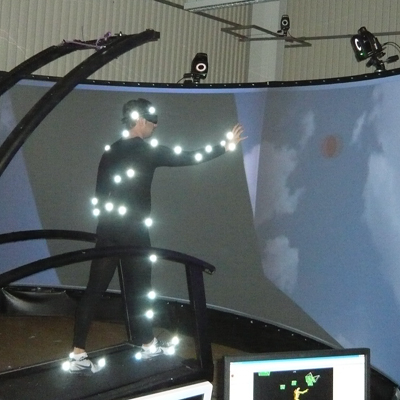
AMARSi (Adaptive Modular Architectures for Rich Motor Skills)
Motor skills of humans and animals are still utterly astonishing when compared to robots. AMARSi aims at a qualitative jump in robotic motor skills towards biological richness.
Read more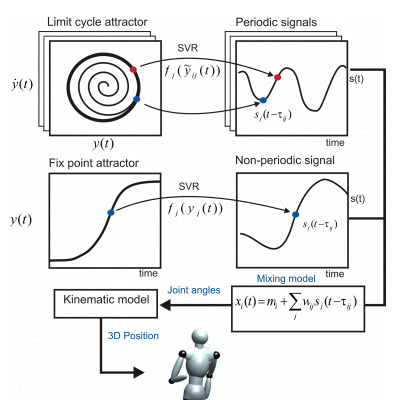
Component-based Trajectory Models for Human Character Animation
The efficient parameterization of complex human movements is a core problem of modern computer animation. For the synthesis of animations with a high degree of realism learning-based approaches have become increasingly popular.
Read more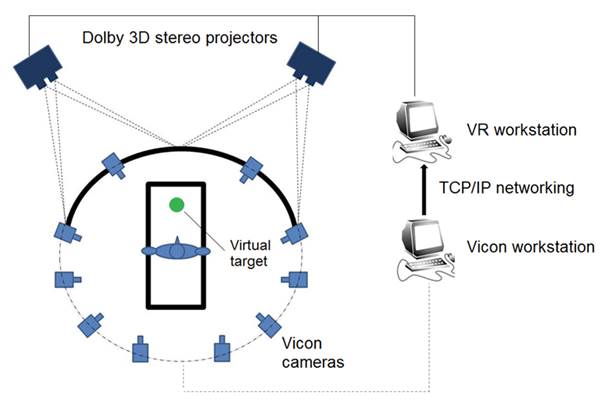
Interaction between periodic and non-periodic kinematic motion primitives
In order to provide a highly controlled setup for the recording of arm movements that are coordinated with walking movements, we have developed a novel virtual reality setup combining motion capture using a VICON system and stereoscopic presentation using a setup with Dolby 3D stereo projectors.
Read more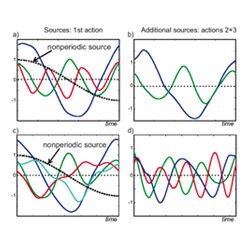
Online motion synthesis by networks of learned dynamic primitives for humanoid robots
Sequential goal-directed full-body motion is a challenging task for humanoid robots. An example is the coordination of bipedal walking with fast upper body movements.
Read more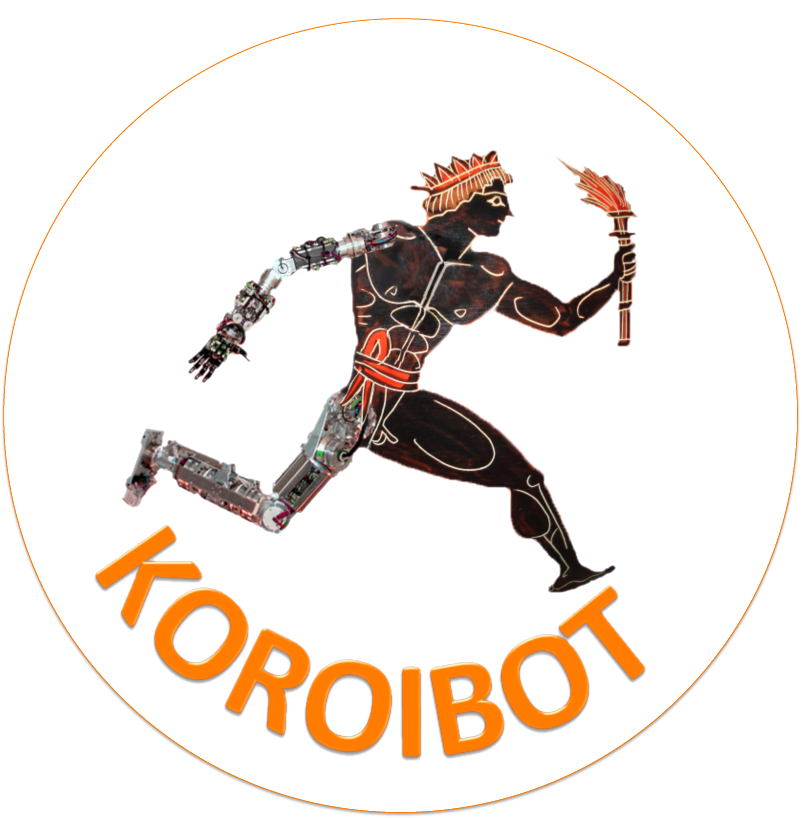
Synthesis of complex locomotion behavior for humanoid robots based on biological principles
Locomotion in complex situations is a difficult problem in motor control that is unresolved for humanoid robots. We investigate and model how the locomotion behavior of humans is organized for complex locomotion tasks and try to transfer relevant control principles, especially at the level of cognitive control, to humaoid robots. This work is realized within the EC FP7 project KOIROBOT.
Read more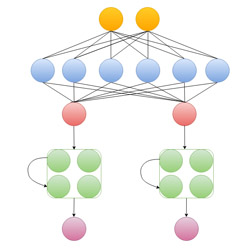
Learning Hierarchical Models for Motor Control
There is strong evidence that the animal Motor Control System is hierarchically organized into highly-interacting specialized subnetworks. In our lab, we combine methods from System Identification Theory and Machine Learning to automatically identify such modules.
Read morePublications
Neural encoding of biomechanically (im)possible human movements in occipitotemporal cortex
Understanding how the human brain processes body movements is essential for clarifying the mechanisms underlying social cognition and interaction. This study investigates the encoding of biomechanically possible and impossible body movements in occipitotemporal cortex using ultra-high field 7Tesla fMRI. By predicting the response of single voxels to impossible/possible movements using a computational modelling approach, our findings demonstrate that a combination of postural, biomechanical, and categorical features significantly predicts neural responses in the ventral visual cortex, particularly within the extrastriate body area (EBA), underscoring the brain{\textquoteright}s sensitivity to biomechanical plausibility. Lastly, these findings highlight the functional heterogeneity of EBA, with specific regions (middle/superior occipital gyri) focusing on detailed biomechanical features and anterior regions (lateral occipital sulcus and inferior temporal gyrus) integrating more abstract, categorical information.Competing Interest StatementThe authors have declared no competing interest.
Human arm redundancy: a new approach for the inverse kinematics problem
The inverse kinematics (IK) problem addresses how both humans and robotic systems coordinate movement to resolve redundancy, as in the case of arm reaching where more degrees of freedom are available at the joint versus hand level. This work focuses on which coordinate frames best represent human movements, enabling the motor system to solve the IK problem in the presence of kinematic redundancies. We used a multi-dimensional sparse source separation method to derive sets of basis (or source) functions for both the task and joint spaces, with joint space represented by either absolute or anatomical joint angles. We assessed the similarities between joint and task sources in each of these joint representations, finding that the time-dependent profiles of the absolute reference frame’s sources show greater similarity to corresponding sources in the task space. This result was found to be statistically significant. Our analysis suggests that the nervous system represents multi-joint arm movements using a limited number of basis functions, allowing for simple transformations between task and joint spaces. Additionally, joint space seems to be represented in an absolute reference frame to simplify the IK transformations, given redundancies. Further studies will assess this finding’s generalizability and implications for neural control of movement.
Parallel Backpropagation for Shared-Feature Visualization
MacAction: Realistic 3D macaque body animation based on multi-camera markerless motion capture
Social interaction is crucial for survival in primates. For the study of social vision in monkeys, highly controllable macaque face avatars have recently been developed, while body avatars with realistic motion do not yet exist. Addressing this gap, we developed a pipeline for three-dimensional motion tracking based on synchronized multi-view video recordings, achieving sufficient accuracy for life-like full-body animation. By exploiting data-driven pose estimation models, we track the complete time course of individual actions using a minimal set of hand-labeled keyframes. Our approach tracks single actions more accurately than existing pose estimation pipelines for behavioral tracking of non-human primates, requiring less data and fewer cameras. This efficiency is also confirmed for a state-of-the-art human benchmark dataset. A behavioral experiment with real macaque monkeys demonstrates that animals perceive the generated animations as similar to genuine videos, and establishes an uncanny valley effect for bodies in monkeys.Competing Interest StatementThe authors have declared no competing interest.
Bodies in motion: Unraveling the distinct roles of motion and shape in dynamic body responses in the temporal cortex
Summary The temporal cortex represents social stimuli, including bodies. We examine and compare the contributions of dynamic and static features to the single-unit responses to moving monkey bodies in and between a patch in the anterior dorsal bank of the superior temporal sulcus (dorsal patch [DP]) and patches in the anterior inferotemporal cortex (ventral patch [VP]), using fMRI guidance in macaques. The response to dynamics varies within both regions, being higher in DP. The dynamic body selectivity of VP neurons correlates with static features derived from convolutional neural networks and motion. DP neurons’ dynamic body selectivity is not predicted by static features but is dominated by motion. Whereas these data support the dominance of motion in the newly proposed “dynamic social perception” stream, they challenge the traditional view that distinguishes DP and VP processing in terms of motion versus static features, underscoring the role of inferotemporal neurons in representing body dynamics.