Description
We investigate the mechanisms of the perception of body movements, and their relationship with motor execution and social signals.
We investigate the mechanisms of the perception of body movements, and their relationship with motor execution and social signals. Our work combines psychophysical experiments and the development of physiologically-inspired neural models in close collaboration with electrophysiologists inside and outside of Tübingen. In addition, exploiting advanced methods from computer animation and Virtual Reality (VR), we investigate the perception of body movements (facial and body expressions) in social communication, and its deficits in psychiatric disorders, such as schizophrenia or autism spectrum disorders. A particular new focus is the study of intentional signals that are conveyed by bodily and facial expressions. For this purpose, we developed highly controlled stimulus sets, exploiting high-end methods from computer graphics. In addition, we develop physiologically-inspired neural models for neural circuits involved in the processing of bodies, actions, and the extraction of intent and social information from visual stimuli.
Researchers
Current Projects
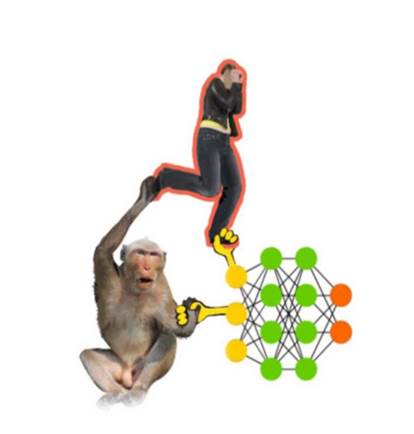
RELEVANCE: How body relevance drives brain organization
Social species, and especially primates, rely heavily on conspecifics for survival. Considerable time is spent watching each other’s behavior for preparing adaptive social responses. The project RELEVANCE aims to understand how the brain evolved special structures to process highly relevant social stimuli, such as bodies and to reveal how social vision sustains adaptive behavior.
Read more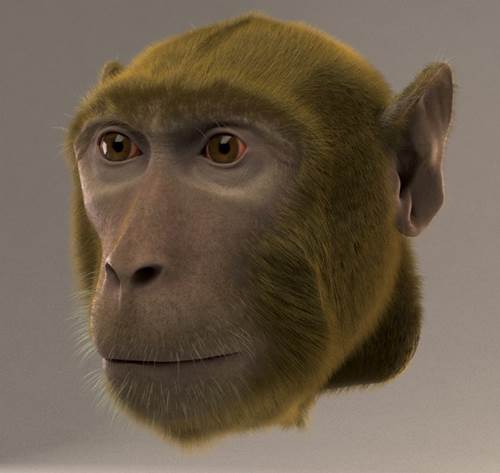
Modelling and Investigation of Facial Expression Perception
Dynamic faces are essential for the communication of humans and non-human primates. However, the exact neural circuits of their processing remain unclear. Based on previous models for cortical neural processes involved for social recognition (of static faces and dynamic bodies), we propose a norm-based mechanism, relying on neurons that represent dierences between the actual facial shape and the neutral facial pose.
Read more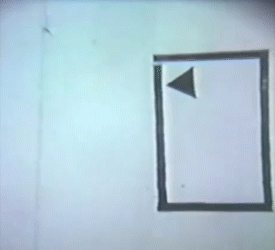
Neural mechanisms underlying the visual analysis of intent
Primates are very efficient in the recognition of intentions from various types of stimuli, involving faces and bodies, but also abstract moving stimuli, such as moving geometrical figures as illustrated in the seminal experiments by Heider and Simmel (1944). How such stimuli are exactly processed and what the underlying neural and computational mechanisms are remains largely unknown.
Read more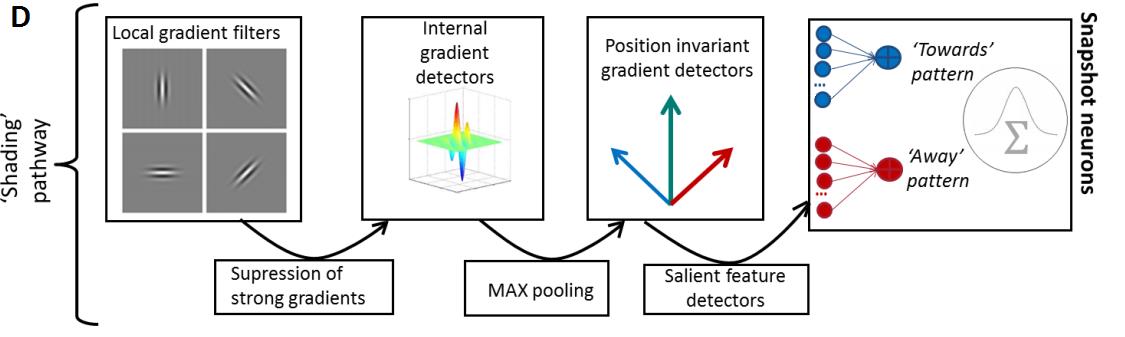
Neural model for shading pathway in biological motion stimuli
Biological motion perception is influenced by shading cues. We study the influence of such cues and develop neural models how the shading cues are integrated with other features in action perception.
Read moreFinished Projects
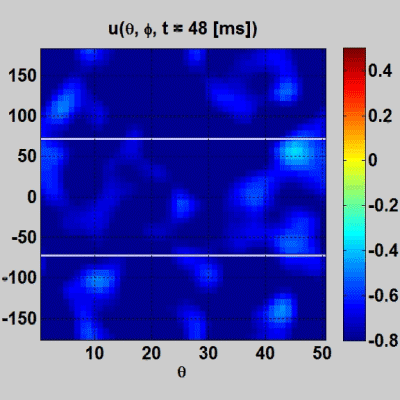
Neural field model for multi-stability in action perception
The perception of body movements integrates information over time. The underlying neural system is nonlinear and is charactrized by a dynamics that supports multi-stable perception. We have investigated multisstable body motion perception and have developed physiologically-inspired neural models that account for the observed psychophysical results.
Read more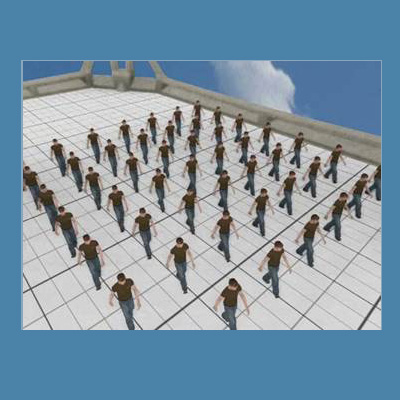
Dynamical Stability and Synchronization in Character Animation
An important domain of the application of dynamical systems in computer animation is the simulation of autonomous and collective behavior of many characters, e.g. in crowd animation.
Read more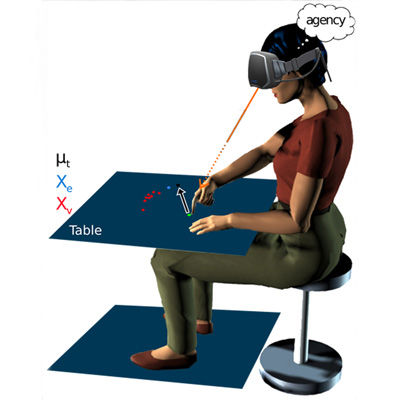
Neural representations of sensory predictions for perception and action
Attribution of percepts to consequences of own actions depends on the consistency between internally predicted and actual visual signals. However, is the attribution of agency rather a binary decision ('I did, or did not cause the visual consequences of the action'), or is this process based on a more gradual attribution of the degree of agency? Both alternatives result in different behaviors of causal inference models, which we try to distinguish by model comparison.
Read more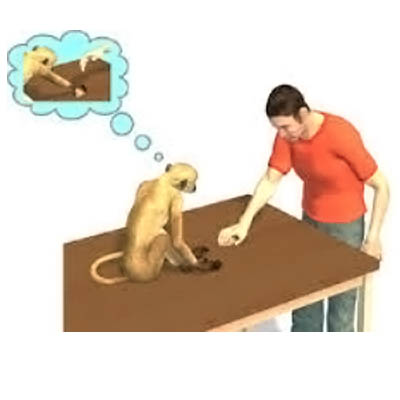
Neuralphysiologically-inspired models of visual action perception and the perception of causality
The recognition of goal-directed actions is a challenging problem in vision research and requires the recognition not only of the movement of amd effector(e.g. the hand) but also the processing its relationship to goal objects, such as a grasped piece of food. In close collaborations with electrophysiologists, we develop models for the neural circuits in cortex that underly this visual function. These models also account for several properties of 'mirror neurons', and for the processing of stimuli (like the one shown in the icon) that suggest causal interactions between objects. In addition, we studied psychophysically the interaction between action observation and exertion using VR methods.
Read more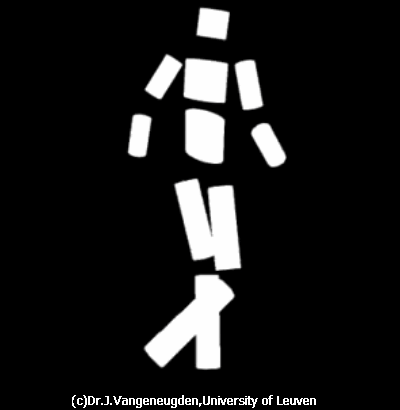
Neurodynamic model for multi-stability in action perception
Action perception is related to interesting dynamical phenomena, such as multi-stability and adaptation. The stimulus shown in this demo is bistable and can be seen as walking obliquely coming out or going into the image plane. Such multistability and associated spontaneous perceptual switches result form the dynamics of the neural representation of perceived actions. We investigate this dynamics pasychophysically and model it using neural network models.
Read more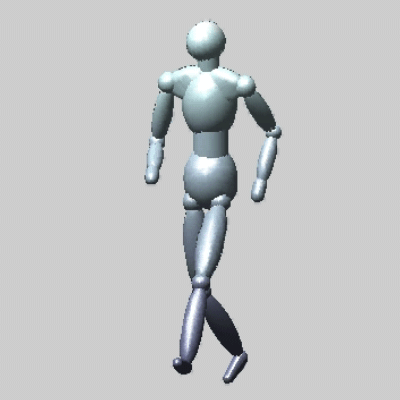
Processing of emotional body expressions in health and disease
Body movements are an important source of information about the emotion of others. The perception of emotional body expressions is impaired in different psychiatric diseases. We have developed methods to generate emotional body motion srimuli with highly-controlled properties, and we exploitz them to study emotion perception in neurologiocal and psychiatric patients.
Read more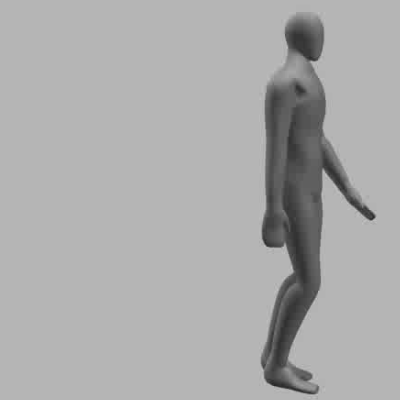
Production and perception of interactive emotional body expressions
A substantial amount of research has addressed the expression and perception of emotions with human faces. Body movements likely also contribute to our expression of emotions. However, this topic has received much less research interest so far. We use techniques from machine learning to synthesize highly-controlled emotional body movements and use them to study the perception and the neural mechanisms of the perception of emotion from bodily emotion expression.
Read more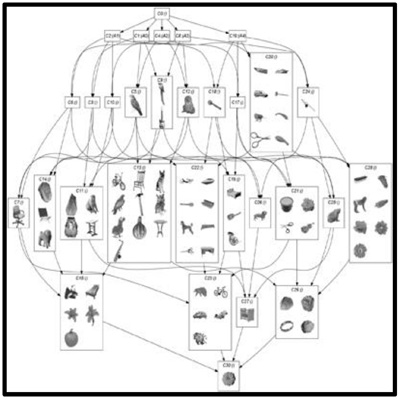
Understanding the semantic structure of the neural code with Formal Concept Analysis
Mammalian brains consist of billions of neurons, each capable of independent electrical activity. From an information-theoretic perspective, the patterns of activation of these neurons can be understood as the codewords comprising the neural code. The neural code describes which pattern of activity corresponds to what information item. We are interested in the structure of the neural code.
Read morePublications
Keypoint-based modeling reveals fine-grained body pose tuning in superior temporal sulcus neurons
Understanding how the human brain processes body movements is essential for clarifying the mechanisms underlying social cognition and interaction. This study investigates the encoding of biomechanically possible and impossible body movements in occipitotemporal cortex using ultra-high field 7Tesla fMRI. By predicting the response of single voxels to impossible/possible movements using a computational modelling approach, our findings demonstrate that a combination of postural, biomechanical, and categorical features significantly predicts neural responses in the ventral visual cortex, particularly within the extrastriate body area (EBA), underscoring the brain{\textquoteright}s sensitivity to biomechanical plausibility. Lastly, these findings highlight the functional heterogeneity of EBA, with specific regions (middle/superior occipital gyri) focusing on detailed biomechanical features and anterior regions (lateral occipital sulcus and inferior temporal gyrus) integrating more abstract, categorical information.Competing Interest StatementThe authors have declared no competing interest.
Pose and shape reconstruction of nonhuman primates from images for studying social perception
The neural and computational mechanisms of the visual encoding of body pose and motion remain poorly understood. One important obstacle in their investigation is the generation of highly controlled stimuli with exactly specified form and motion parameters. Avatars are ideal for this purpose, but for nonhuman species the generation of appropriate motion and shape data is extremely costly, where video-based methods often are not accurate enough to generate convincing 3D animations with highly specified parameters. METHODS: Based on a photorealistic 3D model for macaque monkeys, which we have developed recently, we propose a method that adjusts this model automatically to other nonhuman primate shapes, requiring only a small number of photographs and hand-labeled keypoints for that species. The resulting 3D model allows to generate highly realistic animations with different primate species, combining the same motion with different body shapes. Our method is based on an algorithm that deforms a polygon mesh of a macaque model with 10,632 vertices with an underlying rig of 115 joints automatically, matching the silhouettes of the animals and a small number of specified key points in the example pictures. Optimization is based on a composite error function that integrates terms for matching quality of the silhouettes, keypoints, and bone length, and for minimizing local surface deformation. RRSULTS: We demonstrate the efficiency of the method for several monkey and ape species. In addition, we are presently investigating in a psychophysical experiment how the body shape of different primate species interacts with the categorization of body movements of humans and non-human primates in human perception. CONCLUSION: Using modern computer graphics methods, highly realistic and well-controlled body motion stimuli can be generated from small numbers of photographs, allowing to study how species-specific motion and body shape interact in visual body motion perception. Acknowledgements: ERC 2019-SyG-RELEVANCE-856495; SSTeP-KiZ BMG: ZMWI1-2520DAT700.
Modeling Action-Perception Coupling with Reciprocally Connected Neural Fields
Register and CLS tokens yield a decoupling of local and global features in large ViTs
Recent work has shown that the attention maps of the widely popular DINOv2 model exhibit artifacts, which hurt both model interpretability and performance on dense image tasks. These artifacts emerge due to the model repurposing patch tokens with redundant local information for the storage of global image information. To address this problem, additional register tokens have been incorporated in which the model can store such information instead. We carefully examine the influence of these register tokens on the relationship between global and local image features, showing that while register tokens yield cleaner attention maps, these maps do not accurately reflect the integration of local image information in large models. Instead, global information is dominated by information extracted from register tokens, leading to a disconnect between local and global features. Inspired by these findings, we show that the CLS token itself, which can be interpreted as a register, leads to a very similar phenomenon in models without explicit register tokens. Our work shows that care must be taken when interpreting attention maps of large ViTs. Further, by clearly attributing the faulty behaviour to register and CLS tokens, we show a path towards more interpretable vision models.
Predictive Features in Deep Neural Network Models of Macaque Body Patch Selectivity
Previous work has shown that neurons from body patches in macaque superior temporal sulcus (STS) respond selectively to images of bodies. However, the visual features leading to this body selectivity remain unclear. METHODS: We conducted experiments using 720 stimuli presenting a monkey avatar in various poses and viewpoints. Spiking activity was recorded from mid-STS (MSB) and anterior-STS (ASB) body patches, previously identified using fMRI. To identify visual features driving the neural responses, we used a model with a deep network as frontend and a linear readout model that was fitted to predict the neuron activities. Computing the gradients of the outputs backwards along the neural network, we identified the image regions that were most influential for the model neuron output. Since previous work suggests that neurons from this area also respond to some extent to images of objects, we used a similar approach to visualize object parts eliciting responses from the model neurons. Based on an object dataset, we identified the shapes that activate each model unit maximally. Computing and combining the pixel-wise gradients of model activations from object and body processing, we were able to identify common visual features driving neural activity in the model. RESULTS: Linear models fit the data well, with mean noise-corrected correlations with neural data of 0.8 in ASB and 0.94 in MSB. Gradient analysis on the body stimuli did not reveal clear preferences of certain body parts and were difficult to interpret visually. However, the joint gradients between objects and bodies traced visually similar features in both images. CONCLUSION: Deep neural networks model STS data well, even though for all tested models, explained variance was substantially lower in the more anterior region. Further work will test if the features that the deep network relies on are also used by body patch neurons.