M. Sc. Lappe, Alexander
Section for Computational Sensomotorics
Department of Cognitive Neurology
Hertie Institute for Clinical Brain Research
Centre for Integrative Neuroscience
University Clinic Tübingen
Otfried-Müller-Str. 25
72076 Tübingen, Germany
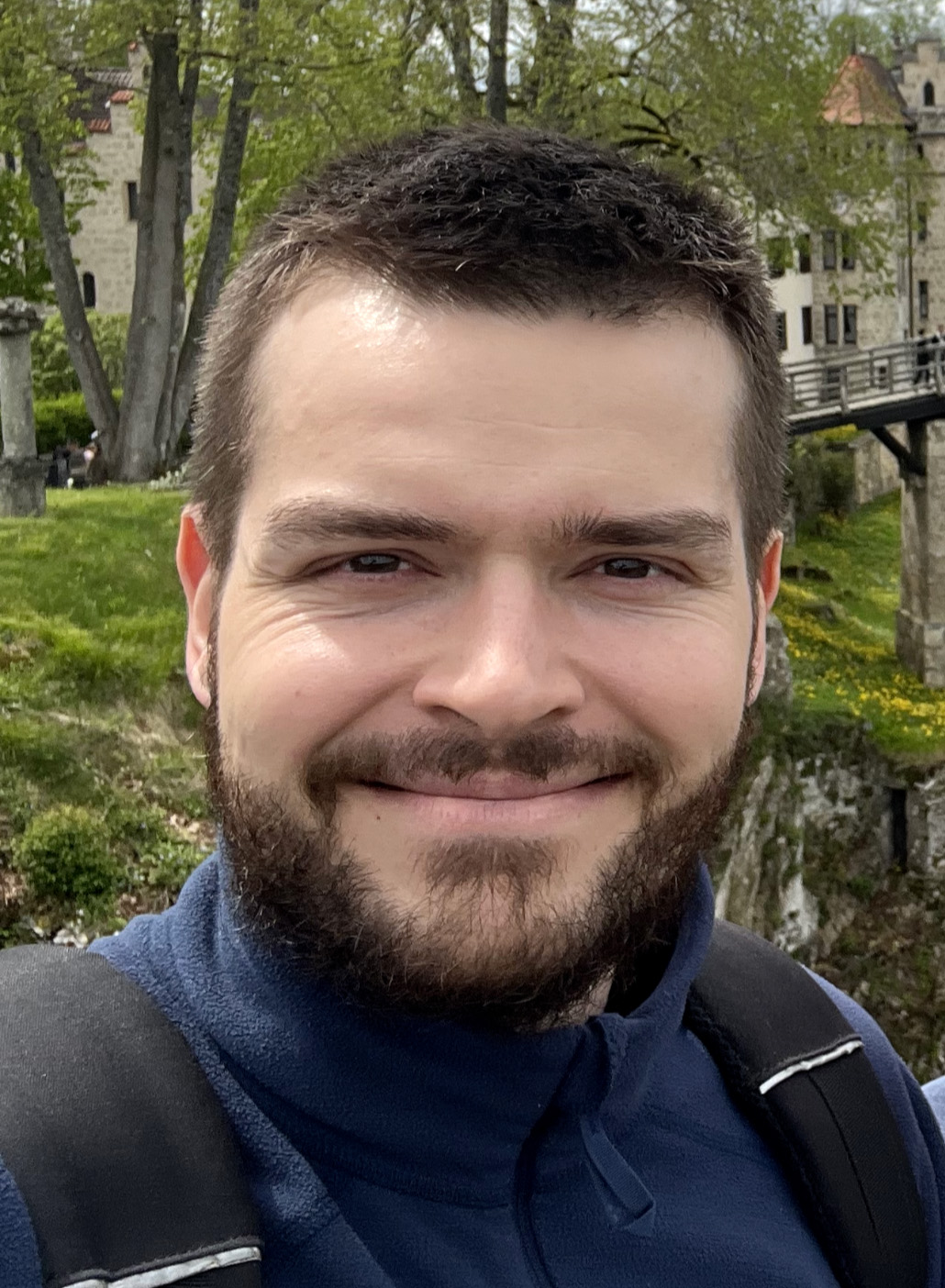