Understanding the semantic structure of the neural code with Formal Concept Analysis
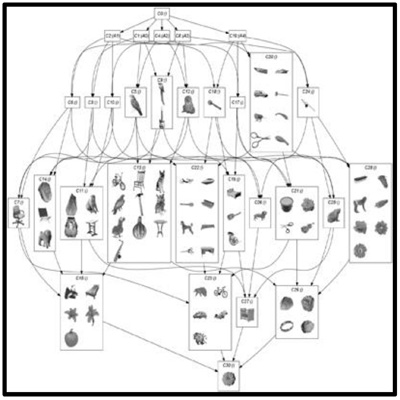
Research Area:
Neural and Computational Principles of Action and Social ProcessingResearchers:
Collaborators:
Dominik EndresDescription:
Mammalian brains consist of billions of neurons, each capable of independent electrical activity. From an information-theoretic perspective, the patterns of activation of these neurons can be understood as the codewords comprising the neural code. The neural code describes which pattern of activity corresponds to what information item. We are interested in the structure of the neural code.
Left: face hierarchy discovered in area STSa of a macaque monkey with Formal Concept Analysis. Neural response specificity increases from top to bottom in this graph. The studied population responds weakly to general face images, more strongly to the caregivers and scientists working with the monkey, and most strongly to images of conspecifics. Right: Indication for a product-of-experts type encoding in high level visual cortex. The parent concepts (on top), which could be labelled 'Face' and 'round' have a common child concept with both characteristics.
Our work on semantic neural coding is concerned with the (high-level) visual system, which is known to encode such information items as the presence of a stimulus object or the value of some stimulus attribute, assuming that each time this item is represented the neural activity will be at least similar.
Neural decoding is the attempt to reconstruct the stimulus (identity) from the observed pattern of activation in a population of neurons. Popular decoding quality measures, such as Fisher's linear discriminant or mutual information capture how accurately a stimulus can be determined from a neural activity pattern. While useful, these measures provide little information about the structure of the neural code, which may hold important clues to the relationships between encoded items.
Understanding how relational, or semantic information is represented in the brain has been an important research direction of neuroscience in the past few years [Kiani et al., 2007; Huth et al. 2012]. From a theoretical perspective, Formal Concept Analysis (FCA) [Ganter & Wille, 1999] has several properties that make it preferrable for uncovering such information: first, FCA is not limited to representing tree-like structures. Second, the structure of the lattice is driven by the data, and not imposed a-priori. If you don't know what FCA is, have a look at the Wikipeda page or at one of the much more in-depth intros found on Uta Priss' FCA page. To our knowledge, we were the first to apply of FCA in a Neuroscience context.
Experimentally, semantic representations have been studied using electrophysiological and brain imaging techniques, specifically electrophysiological single/multi-cell recordings and fMRI BOLD (functional magnetic resonance imaging, blood-oxygenation-level-dependent) responses. The former have the advantage of providing a direct measure of neural electrical activity. However, even with modern techniques it is only possible to record only from a relatively small population of neurons. Another bottleneck in validating the obtained relations is that most of this research is done in animals, which cannot easily be questioned about their semantic perceptions.
Nevertheless, we were able to show [Endres & Földiák 2009] that FCA can reveal interpretable semantic information from electrophysiological data, such as specialization hierarchies, or indications of a feature-based representation. Some examples are shown in the figure above. Furthermore, in [Endres et al., 2010] we studied whether the relational structure of these data is preseved under attribute scaling. We found that the interpretable ordering relations are preserved when the neural responses are mapped onto FCA attributes with higher resolution, indicating that our previous results were not just a result of thresholding noisy neural responses. In these publications, we developed a variant of the Bayesian Bin Classification algorithm [Endres & Földiák, 2008] to convert neural spiketrains Bayes-optimally into attributes (scaled and binary) for FCA.
In [Endres et al., 2012], we investigate whether similar findings can be obtained from BOLD (Blood Oxgenation Level Dependent signal) responses recorded from human subjects. fMRI (functional Magnetic Resonance Imaging) measures BOLD changes which are indirectly related to neuronal activity: neural activity consumes energy and therefore oxygen, leading to increased blood flow near the activated neuron(s), and a change in the ratio between oxygenated and deoxygenated blood. This in turn leads to a change of the magnetic properties of the blood, which can be picked up by fMRI scanners. While the BOLD response provides only an indirect measure of neural activity on a much coarser spatio-temporal scale than electrophysiological recordings (in the order of 25 mm3 per voxel and 1s time resolution for a 3T scanner), it has the advantage that it can be recorded from humans, which can be questioned about their perceptions during or after the experiment, thereby avoiding the need of interpreting animal behavioral responses. Furthermore, the BOLD signal can be recorded from large parts of the brain simultaneously, and not just one, or a few neurons at a time.
We found that, when pre-processed by a sufficiently powerful Bayesian feature extractor which we designed for this purpose, that fMRI signals allow for the decoding of interpretable semantic relationships as well. Furthermore, we were able to validate these relationships by comparing them to the relationships computed from behavioral ratings (similarity and familiarity) performed by the same human subject which was scanned while watching the stimuli (see [Endres et al., 2012] for details). Also worth noting is that the extracted relationships depended on the brain area from which the FCA attributes are derived: when looking at the early visual cortex (V1), we found evidence for shape relations. In contrast, the higher visual area IT yielded attributes which describe properties like 'plant vs. animal' or 'alive vs. man-made' etc.
References:
- Ganter, B. and Wille, R. (1999). Formal Concept Analysis: Mathematical foundations. Springer, Heidelberg.
- Kiani, R. and Esteky, H. and Mirpour, K. and Tanaka, K. (2007). Object category structure in response patterns of neuronal population in monkey inferior temporal cortex. Journal of Neurophysiology 97(6):4296--4309.
- Huth, A.G.and Nishimoto, S. and Vu, A.T. and Gallant, J.L. (2012). A Continuous Semantic Space Describes the Representation of Thousands of Object and Action Categories across the Human Brain. Neuron 76(6):1210 -- 1224.