Description
We investigate the mechanisms of the perception of body movements, and their relationship with motor execution and social signals.
We investigate the mechanisms of the perception of body movements, and their relationship with motor execution and social signals. Our work combines psychophysical experiments and the development of physiologically-inspired neural models in close collaboration with electrophysiologists inside and outside of Tübingen. In addition, exploiting advanced methods from computer animation and Virtual Reality (VR), we investigate the perception of body movements (facial and body expressions) in social communication, and its deficits in psychiatric disorders, such as schizophrenia or autism spectrum disorders. A particular new focus is the study of intentional signals that are conveyed by bodily and facial expressions. For this purpose, we developed highly controlled stimulus sets, exploiting high-end methods from computer graphics. In addition, we develop physiologically-inspired neural models for neural circuits involved in the processing of bodies, actions, and the extraction of intent and social information from visual stimuli.
Researchers
Current Projects
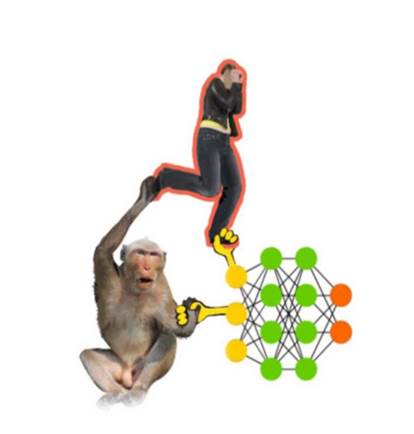
RELEVANCE: How body relevance drives brain organization
Social species, and especially primates, rely heavily on conspecifics for survival. Considerable time is spent watching each other’s behavior for preparing adaptive social responses. The project RELEVANCE aims to understand how the brain evolved special structures to process highly relevant social stimuli, such as bodies and to reveal how social vision sustains adaptive behavior.
Read more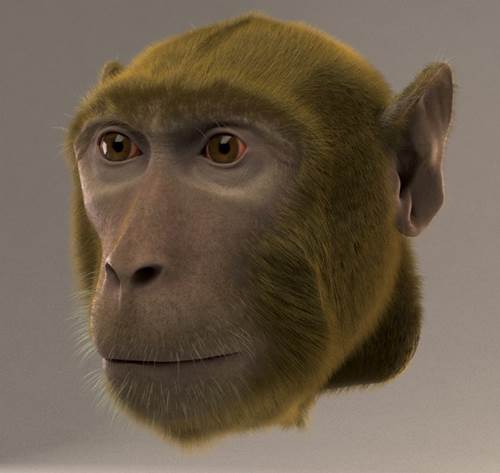
Modelling and Investigation of Facial Expression Perception
Dynamic faces are essential for the communication of humans and non-human primates. However, the exact neural circuits of their processing remain unclear. Based on previous models for cortical neural processes involved for social recognition (of static faces and dynamic bodies), we propose a norm-based mechanism, relying on neurons that represent dierences between the actual facial shape and the neutral facial pose.
Read more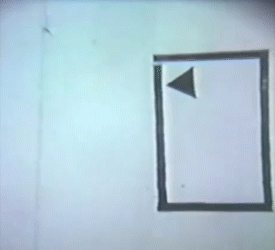
Neural mechanisms underlying the visual analysis of intent
Primates are very efficient in the recognition of intentions from various types of stimuli, involving faces and bodies, but also abstract moving stimuli, such as moving geometrical figures as illustrated in the seminal experiments by Heider and Simmel (1944). How such stimuli are exactly processed and what the underlying neural and computational mechanisms are remains largely unknown.
Read more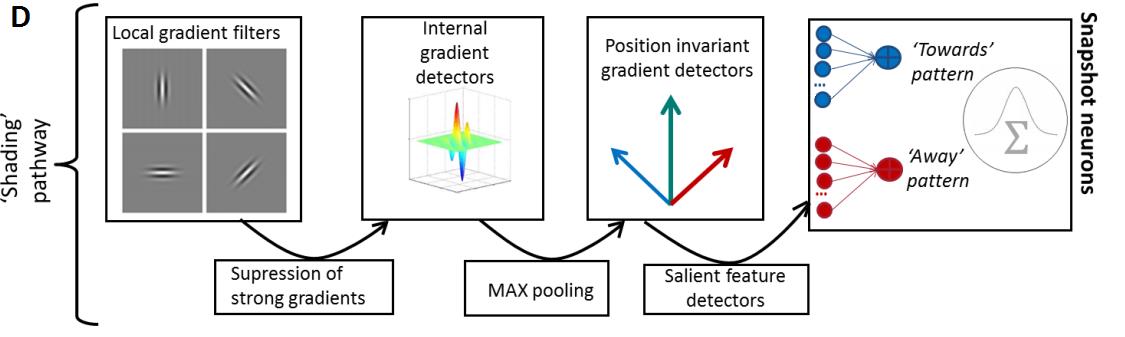
Neural model for shading pathway in biological motion stimuli
Biological motion perception is influenced by shading cues. We study the influence of such cues and develop neural models how the shading cues are integrated with other features in action perception.
Read moreFinished Projects
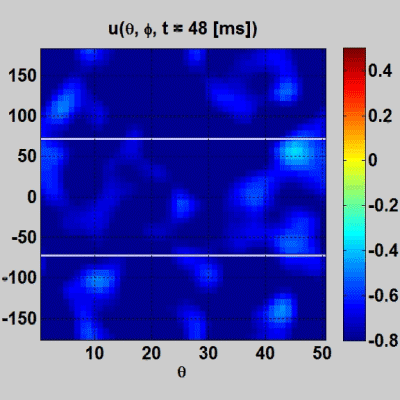
Neural field model for multi-stability in action perception
The perception of body movements integrates information over time. The underlying neural system is nonlinear and is charactrized by a dynamics that supports multi-stable perception. We have investigated multisstable body motion perception and have developed physiologically-inspired neural models that account for the observed psychophysical results.
Read more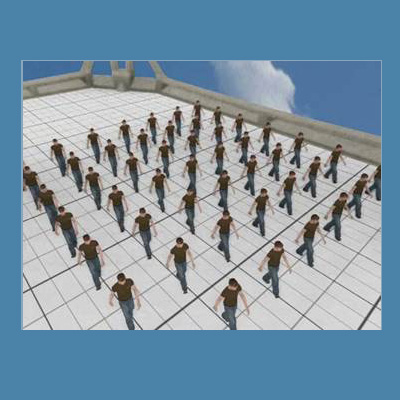
Dynamical Stability and Synchronization in Character Animation
An important domain of the application of dynamical systems in computer animation is the simulation of autonomous and collective behavior of many characters, e.g. in crowd animation.
Read more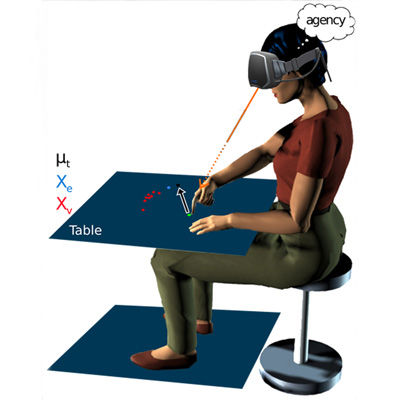
Neural representations of sensory predictions for perception and action
Attribution of percepts to consequences of own actions depends on the consistency between internally predicted and actual visual signals. However, is the attribution of agency rather a binary decision ('I did, or did not cause the visual consequences of the action'), or is this process based on a more gradual attribution of the degree of agency? Both alternatives result in different behaviors of causal inference models, which we try to distinguish by model comparison.
Read more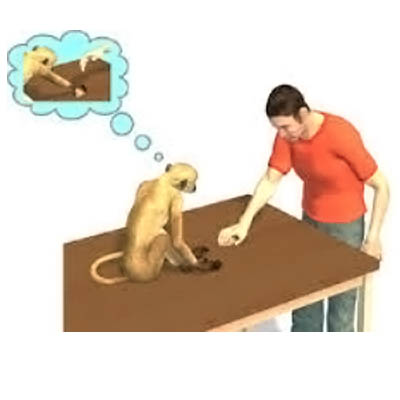
Neuralphysiologically-inspired models of visual action perception and the perception of causality
The recognition of goal-directed actions is a challenging problem in vision research and requires the recognition not only of the movement of amd effector(e.g. the hand) but also the processing its relationship to goal objects, such as a grasped piece of food. In close collaborations with electrophysiologists, we develop models for the neural circuits in cortex that underly this visual function. These models also account for several properties of 'mirror neurons', and for the processing of stimuli (like the one shown in the icon) that suggest causal interactions between objects. In addition, we studied psychophysically the interaction between action observation and exertion using VR methods.
Read more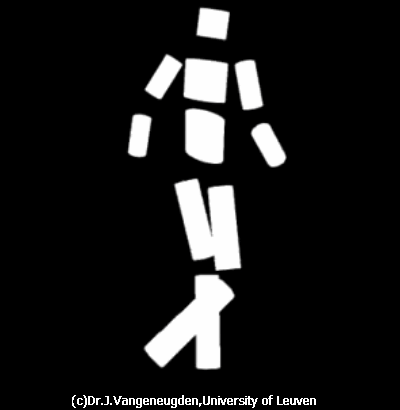
Neurodynamic model for multi-stability in action perception
Action perception is related to interesting dynamical phenomena, such as multi-stability and adaptation. The stimulus shown in this demo is bistable and can be seen as walking obliquely coming out or going into the image plane. Such multistability and associated spontaneous perceptual switches result form the dynamics of the neural representation of perceived actions. We investigate this dynamics pasychophysically and model it using neural network models.
Read more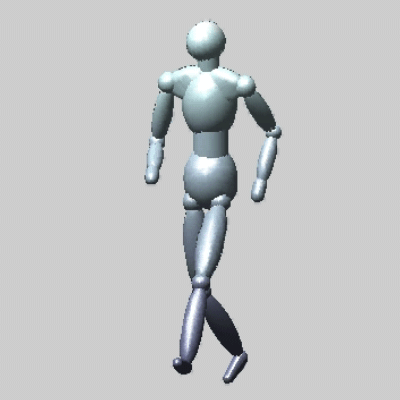
Processing of emotional body expressions in health and disease
Body movements are an important source of information about the emotion of others. The perception of emotional body expressions is impaired in different psychiatric diseases. We have developed methods to generate emotional body motion srimuli with highly-controlled properties, and we exploitz them to study emotion perception in neurologiocal and psychiatric patients.
Read more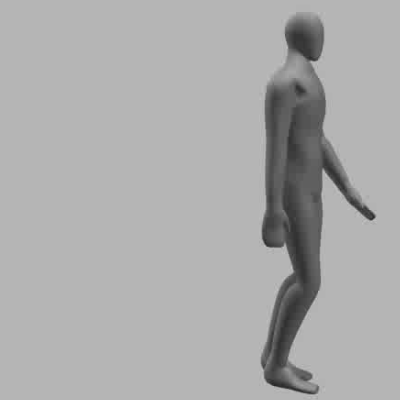
Production and perception of interactive emotional body expressions
A substantial amount of research has addressed the expression and perception of emotions with human faces. Body movements likely also contribute to our expression of emotions. However, this topic has received much less research interest so far. We use techniques from machine learning to synthesize highly-controlled emotional body movements and use them to study the perception and the neural mechanisms of the perception of emotion from bodily emotion expression.
Read more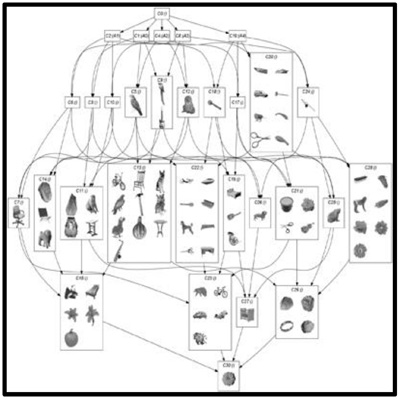
Understanding the semantic structure of the neural code with Formal Concept Analysis
Mammalian brains consist of billions of neurons, each capable of independent electrical activity. From an information-theoretic perspective, the patterns of activation of these neurons can be understood as the codewords comprising the neural code. The neural code describes which pattern of activity corresponds to what information item. We are interested in the structure of the neural code.
Read morePublications
Keypoint-based modeling reveals fine-grained body pose tuning in superior temporal sulcus neurons
Understanding how the human brain processes body movements is essential for clarifying the mechanisms underlying social cognition and interaction. This study investigates the encoding of biomechanically possible and impossible body movements in occipitotemporal cortex using ultra-high field 7Tesla fMRI. By predicting the response of single voxels to impossible/possible movements using a computational modelling approach, our findings demonstrate that a combination of postural, biomechanical, and categorical features significantly predicts neural responses in the ventral visual cortex, particularly within the extrastriate body area (EBA), underscoring the brain{\textquoteright}s sensitivity to biomechanical plausibility. Lastly, these findings highlight the functional heterogeneity of EBA, with specific regions (middle/superior occipital gyri) focusing on detailed biomechanical features and anterior regions (lateral occipital sulcus and inferior temporal gyrus) integrating more abstract, categorical information.Competing Interest StatementThe authors have declared no competing interest.
Pose and shape reconstruction of nonhuman primates from images for studying social perception
The neural and computational mechanisms of the visual encoding of body pose and motion remain poorly understood. One important obstacle in their investigation is the generation of highly controlled stimuli with exactly specified form and motion parameters. Avatars are ideal for this purpose, but for nonhuman species the generation of appropriate motion and shape data is extremely costly, where video-based methods often are not accurate enough to generate convincing 3D animations with highly specified parameters. METHODS: Based on a photorealistic 3D model for macaque monkeys, which we have developed recently, we propose a method that adjusts this model automatically to other nonhuman primate shapes, requiring only a small number of photographs and hand-labeled keypoints for that species. The resulting 3D model allows to generate highly realistic animations with different primate species, combining the same motion with different body shapes. Our method is based on an algorithm that deforms a polygon mesh of a macaque model with 10,632 vertices with an underlying rig of 115 joints automatically, matching the silhouettes of the animals and a small number of specified key points in the example pictures. Optimization is based on a composite error function that integrates terms for matching quality of the silhouettes, keypoints, and bone length, and for minimizing local surface deformation. RRSULTS: We demonstrate the efficiency of the method for several monkey and ape species. In addition, we are presently investigating in a psychophysical experiment how the body shape of different primate species interacts with the categorization of body movements of humans and non-human primates in human perception. CONCLUSION: Using modern computer graphics methods, highly realistic and well-controlled body motion stimuli can be generated from small numbers of photographs, allowing to study how species-specific motion and body shape interact in visual body motion perception. Acknowledgements: ERC 2019-SyG-RELEVANCE-856495; SSTeP-KiZ BMG: ZMWI1-2520DAT700.
Modeling Action-Perception Coupling with Reciprocally Connected Neural Fields
Register and CLS tokens yield a decoupling of local and global features in large ViTs
Recent work has shown that the attention maps of the widely popular DINOv2 model exhibit artifacts, which hurt both model interpretability and performance on dense image tasks. These artifacts emerge due to the model repurposing patch tokens with redundant local information for the storage of global image information. To address this problem, additional register tokens have been incorporated in which the model can store such information instead. We carefully examine the influence of these register tokens on the relationship between global and local image features, showing that while register tokens yield cleaner attention maps, these maps do not accurately reflect the integration of local image information in large models. Instead, global information is dominated by information extracted from register tokens, leading to a disconnect between local and global features. Inspired by these findings, we show that the CLS token itself, which can be interpreted as a register, leads to a very similar phenomenon in models without explicit register tokens. Our work shows that care must be taken when interpreting attention maps of large ViTs. Further, by clearly attributing the faulty behaviour to register and CLS tokens, we show a path towards more interpretable vision models.
Predictive Features in Deep Neural Network Models of Macaque Body Patch Selectivity
Previous work has shown that neurons from body patches in macaque superior temporal sulcus (STS) respond selectively to images of bodies. However, the visual features leading to this body selectivity remain unclear. METHODS: We conducted experiments using 720 stimuli presenting a monkey avatar in various poses and viewpoints. Spiking activity was recorded from mid-STS (MSB) and anterior-STS (ASB) body patches, previously identified using fMRI. To identify visual features driving the neural responses, we used a model with a deep network as frontend and a linear readout model that was fitted to predict the neuron activities. Computing the gradients of the outputs backwards along the neural network, we identified the image regions that were most influential for the model neuron output. Since previous work suggests that neurons from this area also respond to some extent to images of objects, we used a similar approach to visualize object parts eliciting responses from the model neurons. Based on an object dataset, we identified the shapes that activate each model unit maximally. Computing and combining the pixel-wise gradients of model activations from object and body processing, we were able to identify common visual features driving neural activity in the model. RESULTS: Linear models fit the data well, with mean noise-corrected correlations with neural data of 0.8 in ASB and 0.94 in MSB. Gradient analysis on the body stimuli did not reveal clear preferences of certain body parts and were difficult to interpret visually. However, the joint gradients between objects and bodies traced visually similar features in both images. CONCLUSION: Deep neural networks model STS data well, even though for all tested models, explained variance was substantially lower in the more anterior region. Further work will test if the features that the deep network relies on are also used by body patch neurons.
Data-driven Features of Human Body Movements and their Neural Correlate
Macaques show an uncanny valley in body perception
Previous work has shown that neurons from body patches in macaque superior temporal sulcus (STS) respond selectively to images of bodies. However, the visual features leading to this body selectivity remain unclear. METHODS: We conducted experiments using 720 stimuli presenting a monkey avatar in various poses and viewpoints. Spiking activity was recorded from mid-STS (MSB) and anterior-STS (ASB) body patches, previously identified using fMRI. To identify visual features driving the neural responses, we used a model with a deep network as frontend and a linear readout model that was fitted to predict the neuron activities. Computing the gradients of the outputs backwards along the neural network, we identified the image regions that were most influential for the model neuron output. Since previous work suggests that neurons from this area also respond to some extent to images of objects, we used a similar approach to visualize object parts eliciting responses from the model neurons. Based on an object dataset, we identified the shapes that activate each model unit maximally. Computing and combining the pixel-wise gradients of model activations from object and body processing, we were able to identify common visual features driving neural activity in the model. RESULTS: Linear models fit the data well, with mean noise-corrected correlations with neural data of 0.8 in ASB and 0.94 in MSB. Gradient analysis on the body stimuli did not reveal clear preferences of certain body parts and were difficult to interpret visually. However, the joint gradients between objects and bodies traced visually similar features in both images. CONCLUSION: Deep neural networks model STS data well, even though for all tested models, explained variance was substantially lower in the more anterior region. Further work will test if the features that the deep network relies on are also used by body patch neurons.
Neural Encoding of Bodies for Primate Social Perception
Primates, as social beings, have evolved complex brain mechanisms to navigate intricate social environments. This review explores the neural bases of body perception in both human and nonhuman primates, emphasizing the processing of social signals conveyed by body postures, movements, and interactions. Early studies identified selective neural responses to body stimuli in macaques, particularly within and ventral to the superior temporal sulcus (STS). These regions, known as body patches, represent visual features that are present in bodies but do not appear to be semantic body detectors. They provide information about posture and viewpoint of the body. Recent research using dynamic stimuli has expanded the understanding of the body-selective network, highlighting its complexity and the interplay between static and dynamic processing. In humans, body-selective areas such as the extrastriate body area (EBA) and fusiform body area (FBA) have been implicated in the perception of bodies and their interactions. Moreover, studies on social interactions reveal that regions in the human STS are also tuned to the perception of dyadic interactions, suggesting a specialized social lateral pathway. Computational work developed models of body recognition and social interaction, providing insights into the underlying neural mechanisms. Despite advances, significant gaps remain in understanding the neural mechanisms of body perception and social interaction. Overall, this review underscores the importance of integrating findings across species to comprehensively understand the neural foundations of body perception and the interaction between computational modeling and neural recording.
Multi-Domain Norm-referenced Encoding Enables Data Efficient Transfer Learning of Facial Expression Recognition
People can innately recognize human facial expressions in unnatural forms, such as when depicted on the unusual faces drawn in cartoons or when applied to an animal’s features. However, current machine learning algorithms struggle with out-of-domain transfer in facial expression recognition (FER). We propose a biologically-inspired mechanism for such transfer learning, which is based on norm-referenced encoding, where patterns are encoded in terms of difference vectors relative to a domain-specific reference vector. By incorporating domain-specific reference frames, we demonstrate high data efficiency in transfer learning across multiple domains. Our proposed architecture provides an explanation for how the human brain might innately recognize facial expressions on varying head shapes (humans, monkeys, and cartoon avatars) without extensive training. Norm-referenced encoding also allows the intensity of the expression to be read out directly from neural unit activity, similar to face-selective neurons in the brain. Our model achieves a classification accuracy of 92.15% on the FERG dataset with extreme data efficiency. We train our proposed mechanism with only 12 images, including a single image of each class (facial expression) and one image per domain (avatar). In comparison, the authors of the FERG dataset achieved a classification accuracy of 89.02% with their FaceExpr model, which was trained on 43,000 images.
Neurodynamical Model of the Visual Recognition of Dynamic Bodily Actions from Silhouettes
For social species, including primates, the recognition of dynamic body actions is crucial for survival. However, the detailed neural circuitry underlying this process is currently not well understood. In monkeys, body-selective patches in the visual temporal cortex may contribute to this processing. We propose a physiologically-inspired neural model of the visual recognition of body movements, which combines an existing image-computable model (`ShapeComp') that produces high-dimensional shape vectors of object silhouettes, with a neurodynamical model that encodes dynamic image sequences exploiting sequence-selective neural fields. The model successfully classifies videos of body silhouettes performing different actions. At the population level, the model reproduces characteristics of macaque single-unit responses from the rostral dorsal bank of the Superior Temporal Sulcus (Anterior Medial Upper Body (AMUB) patch). In the presence of time gaps in the stimulus videos, the predictions made by the model match the data from real neurons. The underlying neurodynamics can be analyzed by exploiting the framework of neural field dynamics.
Beyond the classic sensory systems: Characteristics of the sense of time of harbor seals (Phoca vitulina) assessed in a visual temporal discrimination and a bisection task
Abstract Beyond the classic sensory systems, the sense of time is most likely involved from foraging to navigation. As a prerequisite for assessing the role time is playing in different behavioral contexts, we further characterized the sense of time of a harbor seal in this study. Supra-second time intervals were presented to the seal in a temporal discrimination and a temporal bisection task. During temporal discrimination, the seal needed to discriminate between a standard time interval (STI) and a longer comparison interval. In the bisection task, the seal learnt to discriminate two STIs. Subsequently, it indicated its subjective perception of test time intervals as resembling either the short or long STI more. The seal, although unexperienced regarding timing experiments, learnt both tasks fast. Depending on task, time interval or duration ratio, it achieved a high temporal sensitivity with Weber fractions ranging from 0.11 to 0.26. In the bisection task, the prerequisites for the Scalar Expectancy Theory including a constant Weber fraction, the bisection point lying close to the geometric mean of the STIs, and no significant influence of the STI pair condition on the probability of a long response were met for STIs with a ratio of 1:2, but not with a ratio of 1:4. In conclusion, the harbor seal's sense of time allows precise and complex judgments of time intervals. Cross-species comparisons suggest that principles commonly found to govern timing performance can also be discerned in harbor seals.
Neural model for the representation of static and dynamic bodies in cortical body patches
Neurophysiologically-inspired computational model of the visual recognition of social behavior and intent
AIMS: Humans recognize social interactions and intentions from videos of moving abstract stimuli, including simple geometric figures (Heider {&} Simmel, 1944). The neural machinery supporting such social interaction perception is completely unclear. Here, we present a physiologically plausible neural model of social interaction recognition that identifies social interactions in videos of simple geometric figures and fully articulating animal avatars, moving in naturalistic environments. METHODS: We generated the trajectories for both geometric and animal avatars using an algorithm based on a dynamical model of human navigation (Hovaidi-Ardestani, et al., 2018, Warren, 2006). Our neural recognition model combines a Deep Neural Network, realizing a shape-recognition pathway (VGG16), with a top-level neural network that integrates RBFs, motion energy detectors, and dynamic neural fields. The model implements robust tracking of interacting agents based on interaction-specific visual features (relative position, speed, acceleration, and orientation). RESULTS: A simple neural classifier, trained to predict social interaction categories from the features extracted by our neural recognition model, makes predictions that resemble those observed in previous psychophysical experiments on social interaction recognition from abstract (Salatiello, et al. 2021) and naturalistic videos. CONCLUSION: The model demonstrates that recognition of social interactions can be achieved by simple physiologically plausible neural mechanisms and makes testable predictions about single-cell and population activity patterns in relevant brain areas. Acknowledgments: ERC 2019-SyG-RELEVANCE-856495, HFSP RGP0036/2016, BMBF FKZ 01GQ1704, SSTeP-KiZ BMG: ZMWI1-2520DAT700, and NVIDIA Corporation.
Physiologically-inspired neural model for anorthoscopic perception
Physiologically-inspired neural model for the visual recognition of dynamic bodies
Neurodynamical model for the visual recognition of dynamic bodies
Neurodynamical model for the visual recognition of dynamic bodies
Neurophysiologically-inspired model for social interactions recognition from abstract and naturalistic stimuli
Physiologically-inspired neural model for social interactions recognition from abstract and naturalistic stimuli
Representation of the observer's predicted outcome value in mirror and nonmirror neurons of macaque F5 ventral premotor cortex
Reactive Hand Movements from Arm Kinematics and EMG Signals Based on Hierarchical Gaussian Process Dynamical Models
Physiologically-inspired Neural Circuits for the Recognition of Dynamic Faces
Physiologically-inspired neural model for the visual recognition of social interactions from abstract and natural stimuli
A naturalistic dynamic monkey head avatar elicits species-typical reactions and overcomes the uncanny valley
Cross-species differences in the perception of dynamic facial expressions
Neural model for the visual recognition of agency and social interaction
Neural model for the visual recognition of social interactions
Learning from the past: A reverberation of past errors in the cerebellar climbing fiber signal
The cerebellum allows us to rapidly adjust motor behavior to the needs of the situation. It is commonly assumed that cerebellum-based motor learning is guided by the difference between the desired and the actual behavior, i.e., by error information. Not only immediate but also future behavior will benefit from an error because it induces lasting changes of parallel fiber synapses on Purkinje cells (PCs), whose output mediates the behavioral adjustments. Olivary climbing fibers, likewise connecting with PCs, are thought to transport information on instant errors needed for the synaptic modification yet not to contribute to error memory. Here, we report work on monkeys tested in a saccadic learning paradigm that challenges this concept. We demonstrate not only a clear complex spikes (CS) signature of the error at the time of its occurrence but also a reverberation of this signature much later, before a new manifestation of the behavior, suitable to improve it.
Adaptation aftereffects reveal representations for encoding of contingent social actions
A hallmark of human social behavior is the effortless ability to relate one’s own actions to that of the interaction partner, e.g., when stretching out one’s arms to catch a tripping child. What are the behavioral properties of the neural substrates that support this indispensable human skill? Here we examined the processes underlying the ability to relate actions to each other, namely the recognition of spatiotemporal contingencies between actions (e.g., a “giving” that is followed by a “taking”). We used a behavioral adaptation paradigm to examine the response properties of perceptual mechanisms at a behavioral level. In contrast to the common view that action-sensitive units are primarily selective for one action (i.e., primary action, e.g., ‘throwing”), we demonstrate that these processes also exhibit sensitivity to a matching contingent action (e.g., “catching”). Control experiments demonstrate that the sensitivity of action recognition processes to contingent actions cannot be explained by lower-level visual features or amodal semantic adaptation. Moreover, we show that action recognition processes are sensitive only to contingent actions, but not to noncontingent actions, demonstrating their selective sensitivity to contingent actions. Our findings show the selective coding mechanism for action contingencies by action-sensitive processes and demonstrate how the representations of individual actions in social interactions can be linked in a unified representation