Smart Eyes: Attending and Recognizing Instances of Salient Events – SEARISE
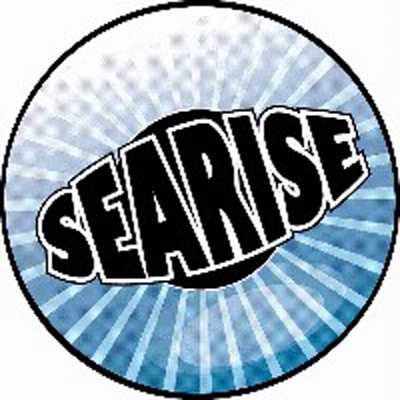
Research Area:
Neural and Computational Principles of Action and Social ProcessingResearchers:
Martin A. Giese;Collaborators:
Dominik EndresDescription:
The SEARISE project develops a trinocular active cognitive vision system, the Smart-Eyes, for detection, tracking and categorization of salient events and behaviours. Unlike other approaches in video surveillance, the system will have human-like capability to learn continuously from the visual input, self-adjust to ever changing visual environment, fixate salient events and follow their motion, categorize salient events dependent on the context. A cyclopean camera performs wide-angle monitoring of the visual field while active binocular stereo cameras will fixate and track salient objects, mimicking a focus of attention that switches between different interesting locations.
The core of this artificial cognitive visual system is a dynamic hierarchical architecture, inspired by computational models of visual processing in the brain. Information processing in Smart-Eyes will be highly efficient due to a multi-scale design: Controlled by the brain-inspired model, the active cameras will provide a multi-scale video record of salient events. The processing will self-organize to adapt to scale variations and to assign the majority of computational resources to the informative parts of the scene.
An assault scence, tracked by the SEARISE system
The Smart-Eyes system will be tested in real-life scenarios featuring the activity of people in different scales. In a long-range distance scenario, the system will be monitoring crowd behaviour of sport fans in a football arena. In a short range scenario, the system will be monitoring the behaviour of small groups of people and single individuals in a subway station. The system's capability for self-adaptation will be specifically demonstrated and quantified compared to systems with 'classical' architecture that are trained once and then used on a set of test scenes.