AMARSi (Adaptive Modular Architectures for Rich Motor Skills)
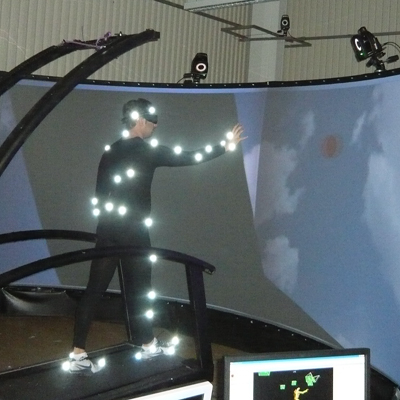
Research Area:
Biomedical and Biologically Motivated Technical ApplicationsResearchers:
Martin A. Giese; Enrico Chiovetto; Albert MukovskiyDescription:
Human and animal movements are still utterly astonishing when compared to robots. The AMARSi Project aims at bridging this gap.
Research includes:
The AMARSi Project has been supported by the FP7 Program, under thematic objective Information and Communication Technology, grant agreement n. 248311
(visit www.amarsi-project.eu for additional information)
Subprojects
Movement primitives are usually assumed to extend over short temporal segments only, which begets the question of how these segments can be identified or defined. We developed a motion segmentation algorithm based on Bayesian Binning.
In order to provide a highly controlled setup for the recording of arm movements that are coordinated with walking movements, we have developed a novel virtual reality setup combining motion capture using a VICON system and stereoscopic presentation using a setup with Dolby 3D stereo projectors.
Complex behavior is thought to be generated by a small number of movement primitives. Multiple definitions of motor primitives have been given in the literature, each one translating into different generative models and different techniques for their identifications.