Cerebellar involvement in the facilitation of action perception by concurrent motor activity
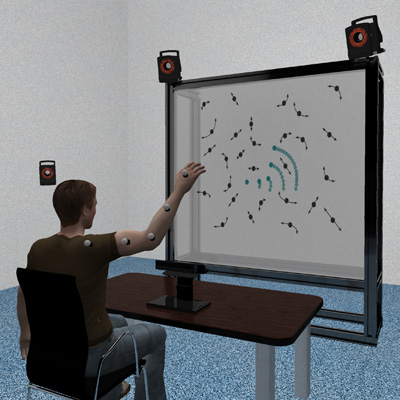
Research Area:
Clinical Movement Control and real-life Behavior Analysis for Assistive SystemsResearchers:
Winfried Ilg; Martin A. Giese;Collaborators:
Dagmar Timmann; Andrea ChristensenDescription:
It has been shown that motor behavior modulates action perception either facilitatory or inhibitory depending on the match of executed and observed action (Christensen et al. 2011). These results have been discussed in light of an internal simulation of observed behavior exploiting motor representations. The cerebellum has been considered as important for underlying internal models. In this study we examine the influence of focal cerebellar lesions on biological motion detection and the action perception coupling. Therefore, we exploit a novel experimental paradigm that combines advanced real-time full-body tracking with the online-generation biological motion stimuli, making visually observed biological motion stimuli directly dependent on the actual motor behavior of the observer.